Machine learning loves data and it produces some interesting results with large volumes of it. Globally, we create an eye-watering 2.5 billion gigabytes of data daily.
There’s a huge amount of data being created and a large portion of this will come from the finance sector. Transactions, outcomes, customers, deals, trading – pretty much everything that happens in the world generates a financial event, and every one of those events creates data.
Machine learning can take huge amounts of data, process it, and help businesses improve their service and performance. For the everyday person, machine learning-powered tech means financial products that are better suited to their needs and ensures they’re always delivered impeccable service. For businesses, machine learning applications can provide a variety of benefits such as better financial visibility and quicker decision making based on real-time information.
Before you get worried about the implications of a technology-powered world of money where the robots take over (we reckon there’s still another 5-10 years before this happens), remember the future isn’t something to dread – it’s something we’re already familiar with.
The global use of point of sale (POS) mobile payments, such as Apple and Google Pay, is forecast to increase to 28% by 2022 according to WorldPay, at which point it will be the second most used POS payment method behind debit cards at 30%.
So, what’s so great about machine learning and how can it be put to good use? Well, there are already instances where improvements are happening for both businesses and individuals. Here are some practical and innovative implementations of machine learning.
1. Chatbots and improved customer service
Amy has arrived at HSBC and she’s brilliant. She talks to every customer who approaches her whatever the time of day or night, and no matter how busy she is. Not only does she help countless numbers of people at once, and without getting bored or tired, she also asks everyone for feedback. This means she also tells HSBC how she might improve her conversations.
Unsurprisingly, Amy isn’t human – although you may not initially realise this when speaking to her. Backed by AI, Amy is a chatbot who uses natural language processing (in pretty much every language of course) across smartphones, computers and any other interactive technology to help with customer inquiries.
She’s not alone in the financial world. The banking app Monzo also uses machine learning to continually improve customer support. In the perfect demonstration of tech and human integration, this solution doesn’t automate everything, instead it will connect users with a live agent when it decides that’s what’s needed. Meanwhile, in the investment management services market, Nutmeg also uses interactive customer-focused technology to keep their costs low and user satisfaction high.
Research from Bloomberg suggests robo-advisors such as Nutmeg will end up managing some £1.5 trillion in assets by 2020 while the Servion Group has said AI will power 95% of all customer interactions within 5 to 10 years. Who knows, if you don’t already you may eventually prefer speaking with a machine rather than a human.
2. Pattern recognition and anomaly detection
As previously mentioned, machine learning loves data and by analysing huge amounts of it the technology can identify trends and patterns. Sometimes these can be used to aid financial decision making or spot when things have gone wrong.
For example, our Fix & Verify functionality within our SaaS platform for:sight uses machine learning to help organisations ensure their VAT data is correct and error-free before submitting. Machine learning technology enables all VAT data to be scrutinised and highlight areas of concern. These anomalies can be manually reviewed and further action taken.
But it doesn’t stop there. Through continued use, Fix & Verify responds to an individual company’s actions and therefore learns how to spot anomalies better. The technology increases in accuracy over time through continued use.
The insurance industry provides another great example of where pattern recognition is being used to business and customer advantage. Insurers work with a huge number of customers, offering diverse policies resulting in multiple outcomes. Despite the level of complexity, allowing the right technology to analyse the data means patterns among customers, claims, policies and more can be identified.
In the US, Lemonade Insurance now uses this functionality to identify and deliver insurance products for homeowners and renters. The decisions it makes are based on behavioural economics – in other words, machine learning decides the risk profile of the applicant based on market experience held within the data and offers the corresponding product. The result? Claims can be resolved and paid within minutes. Minimum paperwork, maximum efficiency.
3. Algorithmic trade aided by machine learning
So how far can machine learning go in the financial sector? Consider this: An effective financial investment is made based on a near infinite number of considerations. Putting the right amount of money in the right area at the right time in order to make a fantastic return requires knowledge of companies, global market trends and much more.
Currently, trading is being supported by algorithms – data and search company Preqin estimates that 1,360 hedge funds currently use computer models to make the majority of their trades.
However, there is a movement towards machine learning and AI taking full control of large investments. Technology is already capable of considering ‘unstructured data’ from diverse sources – such as video reports and audio material – alongside more conventional data held in text and figures. Technology can also make an incredible number of decisions instantly based on that data, which could mean it can manage investments by the minute or even by the second – in any case far more responsively than any human.
However, a fully automated solution may yet be held back by the ultimate complexity of investment decisions. Put simply, making money like this is not as easy as ‘past performance predicts future outcome’ – if it were, maybe we’d all be at it.
Technology and digitalisation will revolutionise the finance industry from top to bottom, from internal processes to external communication. Actually, who are we kidding, it’s already happening. The future is taking shape around us.
As this trend continues it’s worth bearing in mind that machine learning is not just about introducing a great new piece of tech that changes something in the here and now. Perhaps unlike any other tech that might go out of style or be superseded, machine learning will deliver increasing value in the future just through everyday use and that’s something to be excited about in 2020 and beyond.
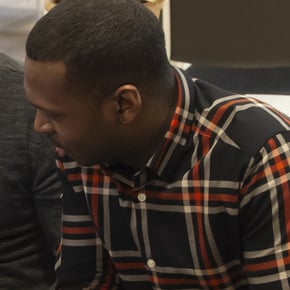